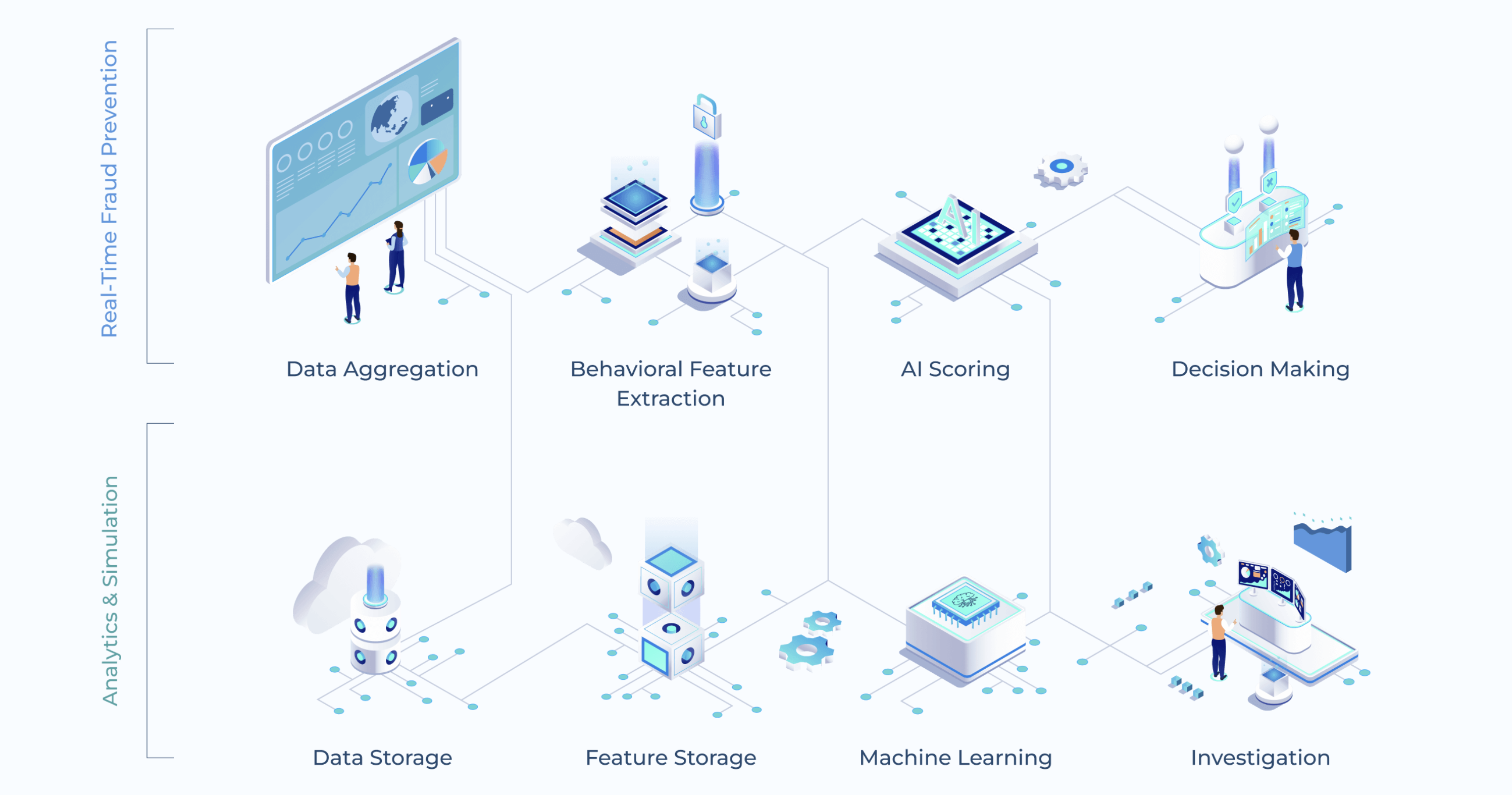
How FraudAverse Works
Customers achieving low fraud with low false positives share the experience that their success does not stem from one single “hot tech” or “wonderful new AI model”. What determines above average business outcome is the result of the clever orchestration of a set of principle functions. (These are the four most important ones.)
Data ingestion
Efficient aggregation of up to hundreds of data streams from all different channels and sources is the key to the generation of well-predicting behavioral features. FraudAverse uses advanced data pipelines to ingest and merge huge amounts of your data into a single condensed analytical datastore.
Feature extraction
Features are extractions of past financial and non-financial activities. Of customers, payment instruments, counterparties, and any entity involved in a payment. The ability to compute behavioral features in real-time that predict the difference of normal to fraudulent transactions is the most crucial factor for low fraud and low false positives.
Artificial intelligence
AI provides the capability to create models through machine learning that can take transaction variables and behavioral features to predict the likelihood of a transaction to be fraudulent. Because different applications require different types of AI, FraudAverse follows an entirely open approach and lets you embed any leading AI.
Decision rules
While AI gets the spotlight in financial crime prevention, a lot of the workload still hinges on decision rules. FraudAverse therefore includes a most flexible rules engine that enables even complex policies to be implemented. It uses machine learning to constantly monitor the effectiveness of the rules and automatically propose improvements to them.