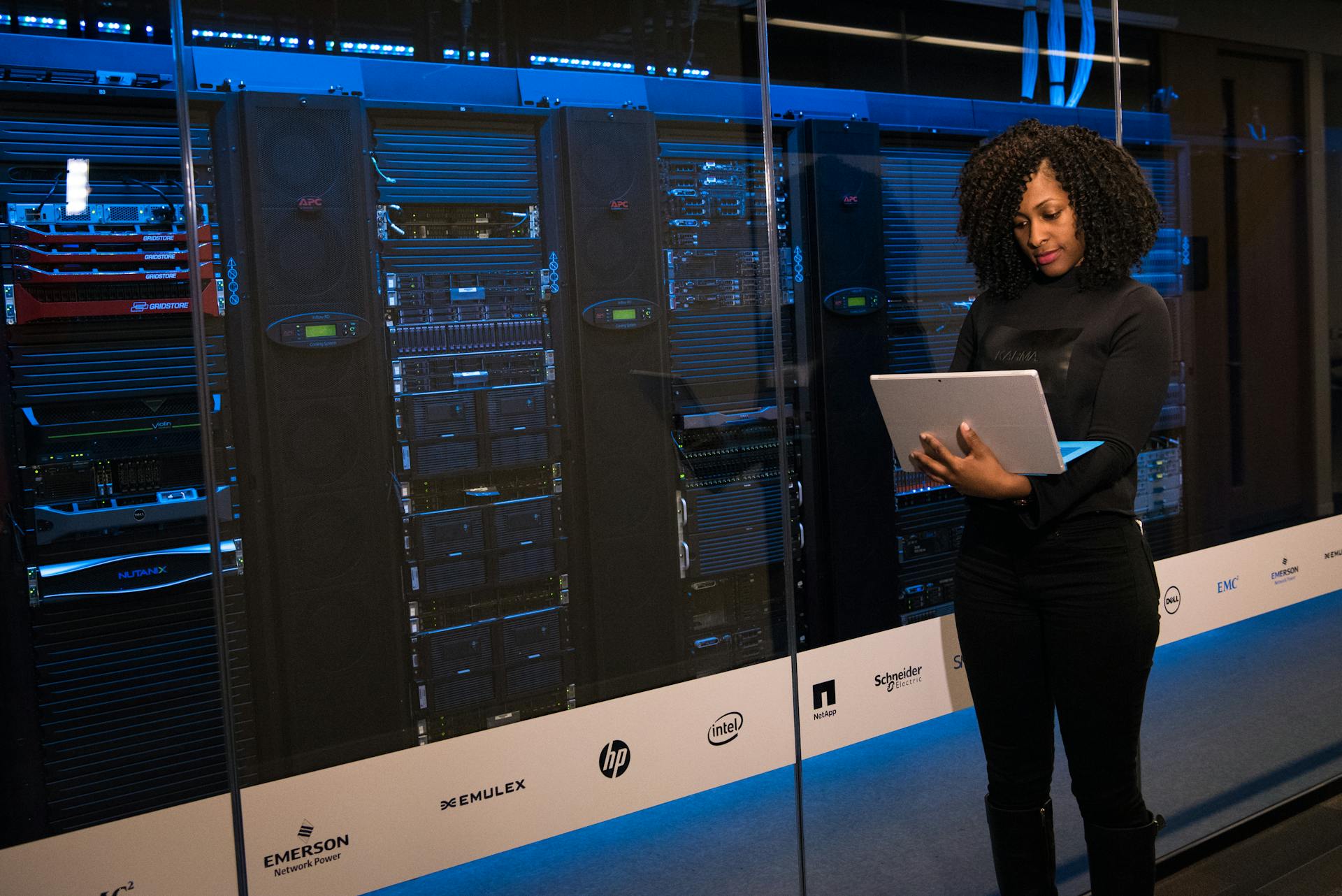
Comparing Performance of Fraud Prevention Systems
We are frequently asked to perform "competitive POCs" by prospective customers to demonstrate how much uplift in fraud detection we can provide by migrating to FraudAverse. In this whitepaper, we are discussing the constraints and limits to this approach.
The easy case
In a "greenfield" situation, where there is no fraud prevention yet in place, a meaningful performance POC for one fraud prevention system is relatively easy to perform. The only prerequisite is that the past payment records are fraud marked. Or that a separate fraud alert file is available that we can merge into the past payment records.
We typically take 3 months of payment records with fraud marks as "training data set" and a subsequent one month of payment records without fraud marks as "verification data set". We then use the training data to create a FraudAverse configuration and to calibrate it to the fraud patterns of our prospective customer. Then we run the verification data through FraudAverse and record the fraud alerts and scores that it generated. Comparing this data to the actual fraud the prospective customer experienced in the verification data period, an accurate result of what results FraudAverse would have achieved can be calculated. This approach is also referred to as "blind test", since for the verification period, we do not know in advance what payments turn out to be fraudulent and which do not.
We typically ask for minimum 10,000 fraudulent payments to be included with the training data set. If there are substantially less fraudulent payments in the training data set, it becomes difficult to create a "stable" fraud prediction model that "generalises" the fraud patterns enough. In other words, if the model is not generalising enough, the criminals would only have to slightly change their fraud schemes to evade detection.
The standard case
Today, most payment processing is already protected by some kind of fraud prevention mechanism. Therefore, the greenfield situation described before has become a rare case. The standard POC case thus is to demonstrate what performance FraudAverse can provide in addition to the existing fraud prevention system.
There is a major flaw of this approach, and that is the statistical bias that is contained in the training/verification data we can get from such a case. It lies in those payments that are rejected/declined by the existing fraud prevention mechanism. These payments are never carried out and therefore there typically is no way of knowing whether they would have turned out to be genuine or not.
This is a particular limit to showcasing FraudAverse's performance as it typically shines not only with fraud loss reduction, but also with a substantial reduction in false positives. In different words, we can never know what high-risk-but-not-fraudulent payments that were rejected by the existing system would have (correctly) not rejected by FraudAverse.
If for example, the customers experiences 10 BP monetary fraud ($1 fraud losses per $1000 turnover), and the existing fraud prevention system catches 4 BP of this at a false/positive rate of 1:20, then the POC could show that FraudAverse would catch 2 BP on top of this (these would be payments that the existing system did not reject) at a false/positive rate of 1:5, this would be a meaningful indicator that replacing the existing system with FraudAverse would most likely increase both the fraud detection rate as well as substantially reduce the false/positives, but there is no way to determine the exact result before a migration.
The best case
If the prospective customer requires precise data on the uplift that FraudAverse brings, he must operate both systems in parallel. And route a certain fraction of the transaction to the existing system, and the remaining ones to FraudAverse. Since FraudAverse is a hosted service, the effort of this is limited since FraudAverse can be provided without any investment in hardware or software. It would only require for the prospective customer to implement the required routing.